A Comprehensive Guide to Choosing the Right AI Solution Architecture for Your Business
Understanding AI Solution Architecture
Choosing the right AI solution architecture is a crucial decision for businesses aiming to leverage artificial intelligence effectively. An AI solution architecture is the structural design that integrates various components of AI technologies, ensuring they work harmoniously to achieve your business goals. Understanding the basics of AI architecture is the first step towards making an informed decision.
The architecture typically involves multiple layers, including data storage, processing, and the integration of machine learning models. Each layer plays a significant role in the overall functionality and efficiency of the AI system. Ensuring these layers are well-coordinated is vital for a robust AI solution.

Identifying Business Needs and Goals
Before diving into the specifics of AI architecture, it's essential to have a clear understanding of your business needs and goals. Identifying these objectives will guide you in selecting the most suitable technologies and frameworks. Ask yourself: What specific problems do we want AI to solve? How will AI integration enhance our current operations?
Once you have a detailed list of objectives, prioritize them. This prioritization will aid in determining which aspects of AI architecture require more focus and investment. Aligning your business strategy with technological capabilities ensures a seamless implementation process.
Scalability and Flexibility
An important consideration in choosing an AI solution architecture is its scalability and flexibility. As your business grows, your AI system should be able to scale without significant redesign or disruptions. Look for architectures that support modular components, allowing for easy upgrades and expansions.
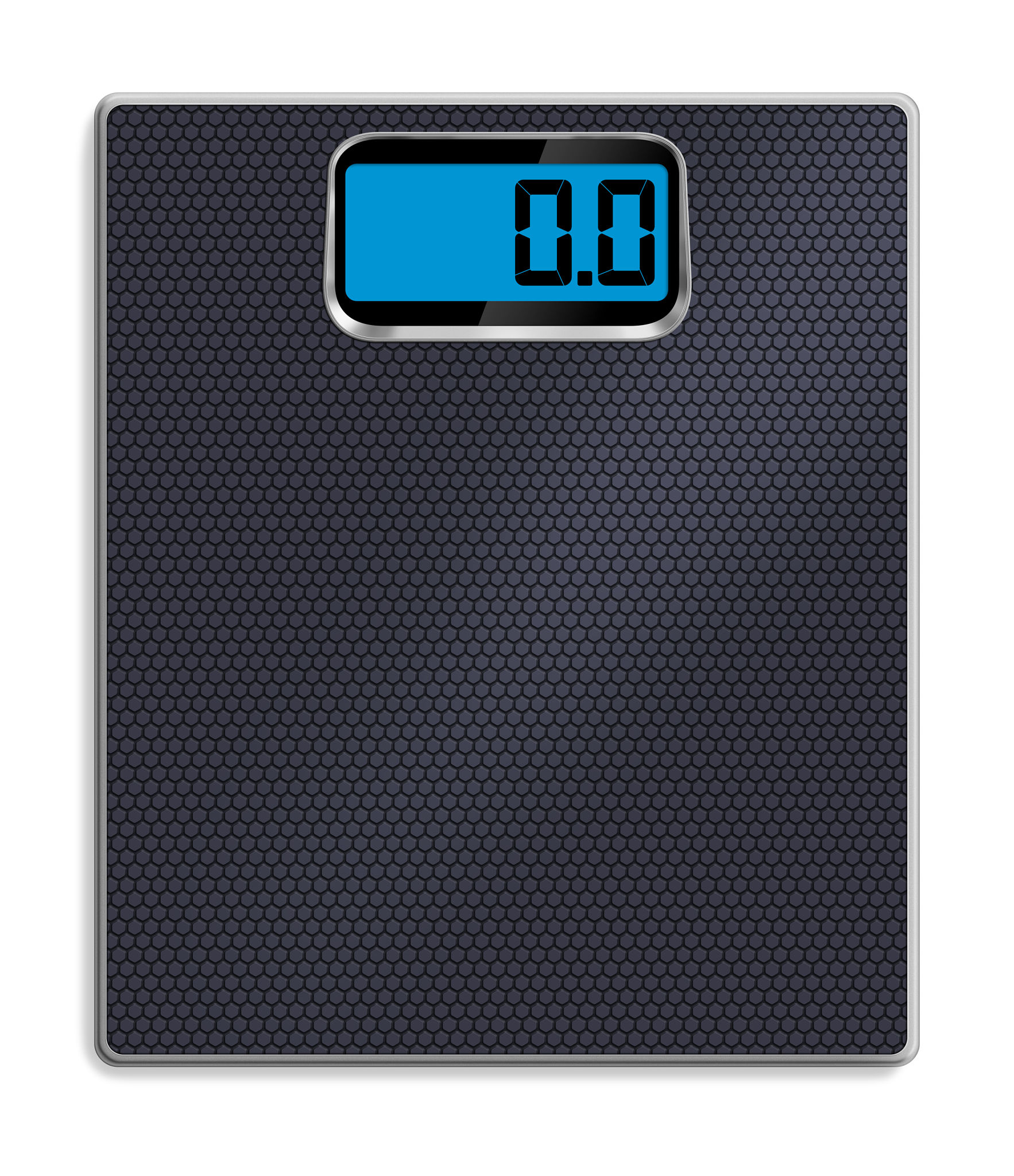
Evaluating Technology Options
With numerous AI technologies available, selecting the right tools is critical. Start by evaluating various platforms and frameworks based on their features, community support, and ease of integration with existing systems. Popular platforms like TensorFlow, PyTorch, and Apache Spark offer diverse functionalities tailored to different business needs.
- TensorFlow: Known for its robustness in handling large-scale machine learning projects.
- PyTorch: Preferred for its flexibility and ease of use in research-based applications.
- Apache Spark: Ideal for big data processing and real-time analytics.
Data Management Strategies
Your chosen AI architecture should include efficient data management strategies. This involves data collection, storage, processing, and security measures. Ensure that the architecture supports seamless data integration from various sources and implements robust security protocols to protect sensitive information.

Integration with Existing Systems
A vital aspect of implementing an AI solution is how well it integrates with your existing systems. An ideal architecture should facilitate smooth communication between new AI components and legacy systems. Consider using APIs and middleware solutions that enable interoperability without extensive reconfiguration.
The ease of integration can significantly impact the overall timeline and cost of your AI project. Choose architectures that offer flexibility in connecting with other business applications to maximize efficiency and performance.
Cost Considerations
Finally, evaluate the cost implications of different AI solution architectures. While some options may require higher initial investments, they might offer long-term benefits in terms of scalability and reduced maintenance costs. Balance your budget constraints with the potential ROI to determine the best fit for your business.

In conclusion, selecting the right AI solution architecture involves careful consideration of various factors, including business goals, scalability, technology options, data management, integration capabilities, and cost. By thoroughly assessing these elements, businesses can implement AI systems that not only meet their current needs but also adapt to future challenges.